陳中興助理教授
陳中興 助理教授 Chung-Hsing Chen
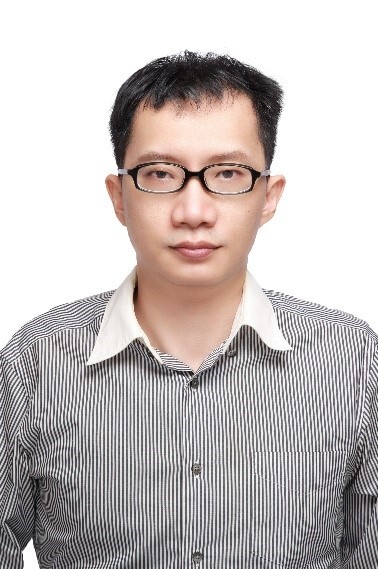
最高學歷:國立中央大學數學所博士
學術專長:生物統計、生物資訊 (詳細如下所示)
開課名稱:線性代數(二)、Python數據科學導論、資料探勘
Office Hour:三 2 - 4
連絡電話:(02)2311-3040 轉分機 1902
E-mail信箱:chchen@uTaipei.edu.tw、chchen@nhri.edu.tw
Last updated:2024 /12 /23
【專長簡介】
作為一名生物統計學家,我專注於利用各種生物醫學數據開發具有臨床應用價值的生物標記,包括遺傳、轉錄/基因表達1-5,11 (例如,微陣列、核糖核酸定序或單細胞核糖核酸定序)、DNA甲基化6-8和RNA干擾9等數據。
這些生物標記的出現使得醫生可以根據每位病患的生物標記特性,精準地選擇合適的藥物。相較於傳統治療方法,「精準醫療」提供了病人更好的治療策略。而在這種「精準醫療」的趨勢下,對於分析這類數據所得到的應用性,提供了巨大的潛力。然而,如何選擇適當的統計方法來分析數據並合理的解釋結果仍存在相當大的不確定性,這使得這些研究的再現性變得困難。為了減少這種不確定性,我將生物醫學數據的分析流程系統地分為四個部分:數據預處理、統計推斷、生物學解釋和考慮在公共衛生方面的潛在影響。使用此流程將能夠更嚴謹的分析數據,提高對這些生物標記的臨床意義的理解,同時也為其他研究者在探索可能的機制時提供更強而有力的證據支持。這些流程不僅需要對統計推論和生物學知識有一定程度的理解,更重要的是與其他領域研究者一起合作的團隊意識。
目前研究的主題是關於癌症治療相關的生物標記,包括急性骨髓性白血病1,4、大腸癌3,8,11與胰臟癌。
此外,我也對流行病學中已知或未知的風險因素如何影響大眾健康感興趣10。政府發布的數據,如癌症登記檔和死因統計檔等,為這些研究提供了寶貴資源。有效的利用這些資源將可以解決重要問題,並成為政府施政的參考。
【Brief Summary of Professional Background】
I am a biostatistician specializing in the development of biomarkers based on various biomedical big data, including genetic data, transcript/gene expression data1-5,11 (for example, microarray, RNA-seq or single cell RNA-seq), DNA methylation data6-8, and RNAi data9.
These data provide the opportunity to identify biomarkers for precision medicine. However, the selection of an appropriate statistical model for analyzing this data remains challenging, making result reproducibility difficult. Specifically, I have systematically divided the analysis pipeline of biomedical big data into four parts: data preprocessing, statistical inferences, biological interpretation, and considerations for public health implications. This pipeline could improve the clinical significance of biomarkers and provide evidence-based support when investigating underlying mechanisms. All of these require a sophisticated understanding of statistical modeling, computational inference, and biological knowledge.
I am interested in identifying and characterizing biomarkers for the diagnosis, prognosis, and therapeutic response in cancer treatments, including chemotherapy, radiation therapy, and immunotherapy, with a specific focus on acute myeloid leukemia1,4, colorectal cancer3,8,11, and pancreatic cancer.
Additionally, I am interested in investigating risk factors in epidemiologic studies10. Government-released data, such as the National Cancer Registry and National Death Registry, among others, provide a valuable resource for initiating a population-based study. These resources would address significant issues and serve as references for government policies.
Selected non-MDPI references (**Co-first author; *Co-corresponding author)
-
Chung-Hsing Chen**, Tsung-Chih Chen**, Ting-Shuan Wu, Tzu-Hung Hsiao, Chi-Ying F. Huang, Po-Liang Cheng, Jia-Rung Tsai, Chieh‐Lin Jerry Teng* (2025) Thyrotropin-releasing hormone and myeloperoxidase within leukemia stem cells increased chemosensitivity in acute myeloid leukemia. Journal of Cellular and Molecular Medicine (Accepted) [link] [SCP2316]
- Yen-Liang Li**, Chung-Hsing Chen**, You-Syuan Lai, Mei-Ren Pan, Wen-Chun Hung* (2024) Increase of blood CSF3R+ myeloid-derived suppressor cell predicts breast cancer recurrence. American Journal of Cancer Research, 14(6): 3171-3185. [link] [SCP1868]
- Yu-Ting Su, Chung-Hsing Chen, Jui-Wen Kang, Hsin-Yu Kuo, Ching-Chieh Yang, Yu-Feng Tian, Cheng-Fa Yeh, Chia-Lin Chou, and Shang-Hung Chen* (2024) Predictive value of FCGBP expression for treatment response and survival in rectal cancer patients undergoing chemoradiotherapy. Aging‑US, DOI: 10.18632/aging.205791. [link]
- Po-Liang Cheng**, Tzu-Hung Hsiao**, Chung-Hsing Chen, Miao-Neng Hung, Pei-Pei Jhan, Li-Wen Lee, Jia-Rung Tsai, Chieh-Lin Jerry Teng* (2023) Chemoresistance in acute myeloid leukemia: An alternative single-cell RNA sequencing approach. Hematological oncology, https://doi.org/10.1002/hon.3129 [link]
- Yen-Liang Li**, Chung-Hsing Chen**, Jing-Yi Chen, You-Syuan Lai, Shao-Chun Wang, Shih Sheng Jiang*, Wen-Chun Hung* (2020) Single-cell analysis reveals immune modulation and metabolic switch in tumor-draining lymph node. Oncoimmunology, DOI: 10.1080/2162402X.2020.1830513. [link]
- Chung-Hsing Chen, Shih Sheng Jiang*, I-Shou Chang, Hui-Ju Wen, Chien-Wen Sun, Shu-Li Wang* (2018) Association between fetal exposure to phthalate endocrine disruptor and genome-wide DNA methylation at birth. Environmental Research, 162: 261-270. [link]
- Ryu Miura, Atsuko Araki, Chihiro Miyashita, Sumitaka Kobayashi, Sachiko Kobayashi, Shu-Li Wang, Chung-Hsing Chen, Kunio Miyake, Mayumi Ishizuka, Yusuke Iwasaki, Yoichi M Ito, Takeo Kubota, Reiko Kishia* (2018) An epigenome-wide study of cord blood DNA methylations in relation to prenatal perfluoroalkyl substance exposure: The Hokkaido study. Environment International, 115: 21-28. [link]
- Chung-Hsing Chen, Shih Sheng Jiang, Ling-Ling Hsieh, Reiping Tang, Chao A. Hsiung, Hui-Ju Tsai*, I-Shou Chang* (2016) DNA methylation identifies loci distinguishing hereditary nonpolyposis colorectal cancer without germline MLH1/MSH2 mutation from sporadic colorectal cancer. Clinical and Translational Gastroenterology, 7(12): e208. [link]
- Chung-Hsing Chen, Wen-Chi Su, Chih-Yu Chen, Jing-Ying Huang, Fang-Yu Tsai, Wen-Chang Wang, Chao A. Hsiung, King-Song Jeng, I-Shou Chang* (2012) A Bayesian measurement error model for two-channel cell-based RNAi data with replicates. Annals of Applied Statistics, 6(1): 356-382. [link]
- Li-Hsin Chien, Chung-Hsing Chen, Tzu-Yu Chen, Gee-Chen Chang, Ying-Huang Tsai, Chin-Fu Hsiao, Kuan-Yu Chen, Wu-Chou Su, Wen-Chang Wang, Ming-Shyan Huang, Yuh-Min Chen, Chih-Yi Chen, Sheng-Kai Liang, Chung-Yu Chen, Chih-Liang Wang, Mei-Hsuan Lee, Ren-Hua Chung, Fang-Yu Tsai, Jia-Wei Hu, Hormuzd A. Katki, Nilanjan Chatterjee, Stephen J. Chanock, Nathaniel Rothman, Qing Lan, Pan-Chyr Yang, Chien-Jen Chen, I-Shou Chang*, Chao A. Hsiung* (2020) Predicting lung cancer occurrence in never-smoking females in Asia: TNSF-SQ, a prediction model. Cancer Epidemiology, Biomarkers & Prevention, 29(2):452-459. [link]
Selected MDPI references (**Co-first author; *Co-corresponding author)
11. Chia-Ming Wu, Chung-Hsing Chen, Kuo-Wang Tsai, Mei-Chen Tan, Fang-Yu Tsai, Shih-Sheng Jiang, Shang-Hung Chen, Wei-Shone Chen, Horng-Dar Wang*, Tze-Sing Huang* (2024) TCF12 and lncRNA MALAT1 cooperatively harness high cyclin D1 but low β-Catenin gene expression to exacerbate colorectal cancer prognosis independently of metastasis. Cells, 13(24):10.3390/cells13242035. [link]